Langning Huo
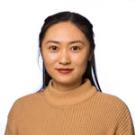
Forskning
2025–2028 STS4ForestStress: Developing Spectral,Temporal, and Spatial Analysis Techniques for Monitoring Forest Stress under Changing Climate, PI, 6.14 million SEK funded by Swedish Research Council Formas.
2025–2027 A co-aligned hyperspectral drone system for improved detection of forest stress, PI, 1.4 million SEK funded by Kempestiftelserna.
2024–2026 Developing remote sensing to map forest stress using data from multiple sensors and platforms, PI, 1.6 million SEK funded by Skogssällskapet.
2024–2026 Data collection for developing remote sensing of forest drought damage, PI, 2.1 million SEK, co-funded by SLU Forest Damage Center, Kempestiftelserna, and Carl Tryggers Stiftelse för vetenskaplig forskning.
2023–2027 Developing remote sensing techniques for monitoring forest damage and disturbance in a changing climate, PI, 2.4 million SEK funded by the SLU Forest Damage Center.
2023–2025 RESDINET, Network for novel remote sensing technologies in forest disturbance ecology, Horizon Europe Framework Programme, Co-PI, coordinator of SLU, In total EUR 1 495 880, shared EUR 383,333.
2022–2024 Developing hyperspectral drone imagery for improved monitoring of forest insect damages, PI, 3.1 million SEK, co-funded by Kempestiftelserna, SLU Forest Damage Center, Hildur & Sven Wingquists stiftelse.
2022–2023 Research exchange for drone-based hyperspectral imagery for early detection of bark beetle infestations, PI, 1.6 million SEK, co-funded by Swedish innovation agency Vinnova, SLU Forest Damage Center, SLU Broderna Edlunds Scholarship.
2020–2022 Improved detection and prediction of spruce bark beetle infestations using multispectral drone images, PI, 2.6 million SEK, co-funded by Stiftenlsen Seydlitz MP bolagen, Stiftelsen Nils och Dorthi Troëdssons forskningsfond, Hildur & Sven Wingquists stiftelse, SLU Forest Damage Center.
2020–2024 Mapping forest parameters and forest damage for sustainable forest management from data fusion of satellite data, MOST/ESA Dragon 5 Cooperation, PI.
Publikationer i urval
1. Huo, L., Koivumäki, N., Näsi, R., & Honkavaara, E. (2025). Sensitivity analysis of the Green Shoulder indices in pre-emergence detection of single trees attacked by European spruce bark beetle. International Journal of Remote Sensing, 1–13.
2. Huo, L., Matsiakh, I., Bohlin, J., & Cleary, M. (2025). Estimation of Tree Vitality Reduced by Pine Needle Disease Using Multispectral Drone Images. Remote Sensing, 17, 271.
3. Bozzini, A., Huo, L., Brugnaro, S., Morgante, G., Persson, H.J., Finozzi, V., Battisti, A., & Faccoli, M. (2025). Multispectral drone images for the early detection of bark beetle infestations: assessment over large forest areas in the Italian South-Eastern Alps. Frontiers in Forests and Global Change, 8, 199.
4. Huo, L., Koivumäki, N., Oliveira, R.A., Hakala, T., Markelin, L., Näsi, R., Suomalainen, J., Polvivaara, A., Junttila, S., & Honkavaara, E. (2024). Bark beetle pre-emergence detection using multi-temporal hyperspectral drone images: Green shoulder indices can indicate subtle tree vitality decline. ISPRS Journal of Photogrammetry and Remote Sensing, 216, 200–216.
5. Huo, L., Persson, H.J., & Lindberg, E. (2024). Analyzing the environmental risk factors of European spruce bark beetle damage at the local scale. European Journal of Forest Research, 137, 675.
6. Persson, H.J., Kärvemo, S., Lindberg, E., Huo, L., (2024). Large-scale wall-to-wall mapping of bark beetle damage and forest practices using the distance red swir index and operational harvester data. Ecological Indicators 162, 112036.
7. Li, N., Huo, L., & Zhang, X. (2024). Using only the red-edge bands is sufficient to detect tree stress: A case study on the early detection of PWD using hyperspectral drone images. Computers and Electronics in Agriculture, 217, 108665.
8. Kärvemo, S.; Huo, L.; Öhrn, P.; Lindberg, E.; Persson, H. J. (2023): Different triggers, different stories: Bark-beetle infestation patterns after storm and drought-induced outbreaks. In Forest Ecology and Management 545 (1), p. 121255. DOI: 10.1016/j.foreco.2023.121255.
9. Huo, L., Lindberg, E., Bohlin, J., & Persson, H.J. (2023). Assessing the detectability of European spruce bark beetle green attack in multispectral drone images with high spatial- and temporal resolutions. Remote Sensing of Environment, 287, 113484. (1% top-cited research paper, Essential Science Indicators)
10. Huo, L., Strengbom, J., Lundmark, T., Westerfelt, P., & Lindberg, E. (2023). Estimating the conservation value of boreal forests using airborne laser scanning. Ecological Indicators, 147, 109946
11. Huo, L., Lindberg, E., & Holmgren, J. (2022). Towards low vegetation identification: A new method for tree crown segmentation from LiDAR data based on a symmetrical structure detection algorithm (SSD). Remote Sensing of Environment, 270, 112857.
12. Yu, R., Huo, L., Huang, H., Yuan, Y., Gao, B., Liu, Y., Yu, L., Li, H., Yang, L., Ren, L., & Luo, Y. (2022). Early detection of pine wilt disease tree candidates using time-series of spectral signatures. Frontiers in Plant Science, 13, 48.
13. Li, N., Huo, L., Zhang, X., (2022). Classification of pine wilt disease at different infection stages by diagnostic hyperspectral bands. Ecological Indicators, 142, 109198.
14. Huo, L., Persson, H., Lindberg, E., (2021). Early detection of forest stress from European spruce bark beetle attack, and a new vegetation index: Normalized Distance Red & SWIR (NDRS). Remote Sensing of Environment, 255, 112240. (1% top-cited research paper, Essential Science Indicators)
15. Huo, L., & Lindberg, E. (2020). Individual tree detection using template matching of multiple rasters derived from multispectral airborne laser scanning data. International Journal of Remote Sensing, 41, 9525–9544.
16. Huo, L., & Zhang, X. (2019). A new method of equiangular sectorial voxelization of single-scan terrestrial laser scanning data and its applications in forest defoliation estimation. ISPRS Journal of Photogrammetry and Remote Sensing, 151, 302–312.
17. Huo, L., Zhang, N., Zhang, X., & Wu, Y. (2019). Tree defoliation classification based on point distribution features derived from single-scan terrestrial laser scanning data. Ecological Indicators, 103, 782–790.